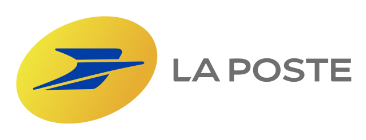
La Poste is a global organization thatspans across retail, banking, logistics, parcel delivery and digital services. The company has operations in 44 countries around the world and generates €26billion annually.
Their project: Use machine learning to better predict delivery time slots. Specifically, predict foreach address, each day, the likely delivery window basedon historical and near real-time data
Their Challenges:
Letters and package delivery operations have much in common.
However, unlike letters, the recipient of a package typically pays for the delivery service in addition to its purchase. For this reason, online shoppers are extremely demanding in terms of speed, convenience, reliability and visibility. But, they are also price sensitive.
Similarly, for merchants, last-mile logistics is a key part of the economic equation, typically accounting for a substantial portion of their shipping costs. It is also a critical factor in the customer experience.
At the time of the project kickoff, the accuracy of La Poste’s estimated delivery time was limited to a half day window. Despite such a conservative time frame, these estimates were consistently inaccurate. As a direct consequence, missed delivery windows were the norm, customer satisfaction suffered, and costs increased as deliveries needed to be rescheduled.
While La Poste maintained an abundance of tracking and tracing data, it struggled to capitalize on the data to tell the whole story. One known issue stemmed from operators scanning many items at once in burst mode. This created downstream analysis problems as the data was difficult to leverage because many items shared the same time and location. Extracting insight was nearly impossible.
In order to improve customer satisfaction and gain an operational advantage, La Poste partnered with Provision.io to add more precision to the delivery windows and improve the delivery rate.
Provision.io’s response:
La Poste’s data science teams were quickly able to leverage the disparate tracking data provided by its own operations and those of its business partners. The key phases of the project and the ultimate success relied upon the team completing the following actions:
- Formulated the problem in business terms
- Translated the business issue into a data science project (scope of analysis, data history,definition of the target)
- Chose the technical metrics suited to the use case, namely the precision rate of the number of packages delivered in the one-hour window
- Set up learning by using a personalized cross validation based on the rounds of the delivery personnel
- Optimized the training pipeline (try more algorithms X feature engineering in less time)
- Used the features selection functionality to speed up the training process by intelligently preselecting the variables relevant to the user’s problem
- Promoted the experience tracking of the different iterations (versioning, …)
” Provision.io’s platform has allowed us to divide the time-to-market of our AI projects by 4.”
Rachid Alili
Chief Data Scientist ot La Poste Group
Benefits for La Poste:
By taking advantage of the simplicity and automation within the platform, the team was able to deploy in 4 weeks as compared to prior initiatives that took at least 6 months.
From an external perspective, the use of this model has led to a substantial improvement in customer satisfaction and a decrease in complaints.
Provision.io delivered a precision of 89%. Alternative solutions using large services team + expensive software licensing were only able to achieve at best a 79% precision level.
Beyond this use case, Provision.io has been instrumental in helping La Poste tackle other key initiatives:
- Optimization of letter carrier rounds,
- Forecasting at 1 month of the number of envelopes / packages delivered
- Detection and extraction of scanned information
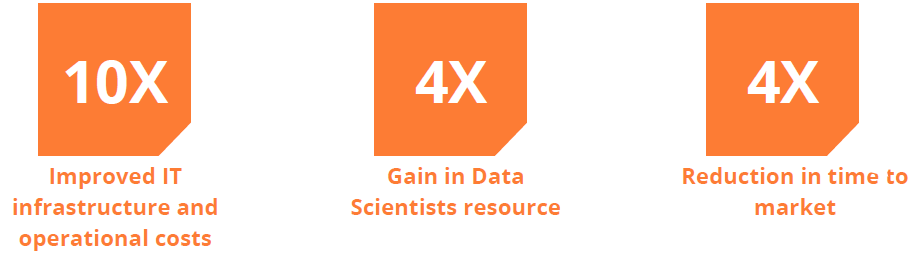